ImagingNotes.com
is available for sale
About ImagingNotes.com
Former domain of a premier publication for commercial, government, and academic remote sensing professionals around the world.
Exclusively on Odys Marketplace
$6,480
What's included:
Domain name ImagingNotes.com
Become the new owner of the domain in less than 24 hours.
Complimentary Logo Design
Save time hiring a designer by using the existing high resolution original artwork, provided for free by Odys Global with your purchase.
Built-In SEO
Save tens of thousands of dollars and hundreds of hours of outreach by tapping into the existing authority backlink profile of the domain.
Free Ownership Transfer
Tech Expert Consulting
100% Secure Payments
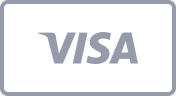

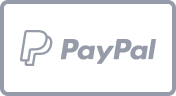

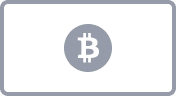
Premium Aged Domain Value
Usually Seen In
Age
Traffic
SEO Metrics
Own this Domain in 3 Easy Steps
With Odys, buying domains is easy and safe. Your dream domain is just a few clicks away.
.1
Buy your Favorite Domain
Choose the domain you want, add it to your cart, and pay with your preferred method.
.2
Transfer it to your Registrar
Follow our instructions to transfer ownership from the current registrar to you.
.3
Get your Brand Assets
Download the available logos and brand assets and start building your dream website.
Trusted by the Top SEO Experts and Entrepreneurs
Rachel Parisi
★ ★ ★ ★ ★
I purchased another three aged domains from Odys in a seamless and painless transaction. John at Odys was super helpful! Odys is my only source for aged domains —you can trust their product.
Stefan
★ ★ ★ ★ ★
Odys is absolutely the best premium domain marketplace in the whole internet space. You will not go wrong with them.
Adam Smith
★ ★ ★ ★ ★
Great domains. Great to deal with. In this arena peace of mind can be difficult to come by, but I always have it with Odys and will continue to use them and recommend them to colleagues and clients.
Brett Helling
★ ★ ★ ★ ★
Great company. Very professional setup, communication, and workflows. I will definitely do business with Odys Global moving forward.
Larrian Gillespie Csi
★ ★ ★ ★ ★
I have bought 2 sites from Odys Global and they have both been of high quality with great backlinks. I have used one as the basis for creating a new site with a great DR and the other is a redirect with again high DR backlinks. Other sites I have looked through have low quality backlinks, mostly spam. I highly recommend this company for reliable sites.
Henry Fox
★ ★ ★ ★ ★
Great company!
Vijai Chandrasekaran
★ ★ ★ ★ ★
I’ve bought over 30 domains from Odys Global in the last two years and I was always very satisfied. Besides great quality, niche-specific auction domains, Alex also helped me a lot with SEO and marketing strategies. Auction domains are not cheap, but quality comes with a price. If you have the budget and a working strategy, these domains will make you serious money.
Keith
★ ★ ★ ★ ★
Earlier this year, I purchased an aged domain from Odys as part of a promo they’re running at the time. It was my first experience with buying an aged domain so I wanted to keep my spend low. I ended up getting a mid level DR domain for a good price. The domain had solid links from niche relevant high authority websites. I used the site as a 301 redirect to a blog I had recently started. Within a few weeks I enjoyed new traffic levels on my existing site. Happy to say that the Odys staff are friendly and helpful and they run a great business that is respected within the industry.